Micro Tells Macro:
Popularity Prediction for Micro-Videos
Abstract
Micro-videos, a new form of user generated contents (UGCs), are gaining increasing enthusiasm. Popular microvideos has profound commercial value in many ways, such as online marketing and brand tracking. In fact, the popularity prediction of traditional UGCs including tweets, web images, and long videos, has achieved well theoretical underpinnings and great practical success. However, little research thus far has been conducted to predict the popularity of the bite-sided videos. Honestly, this task is non-trivial due to these reasons: 1) micro-videos are short in length and of low quality; 2) they can be described by multiple heterogeneous channels, spanning from social, visual, acoustic to textual modalities; and 3) there are no available benchmark dataset and discriminant features that are suitable for this task. Towards this end, in this work, we present a transductive multi-modal learning model. The proposed model is designed to nd the optimal latent common space, unifying and preserving information from dierent modalities, whereby micro-videos can be better represented. This latent space can somehow alleviate the information insucient problem caused by the short nature of micro-videos. In addition, we built a benchmark dataset and extracted a rich set of popularity-oriented features to characterize the popular micro-videos. Extensive experiments have demonstrated the eectiveness of the proposed model. As a side contribution, we have released the dataset, codes and parameters to facilitate other researchers. The framework of our proposed method is shown in Figure 1.
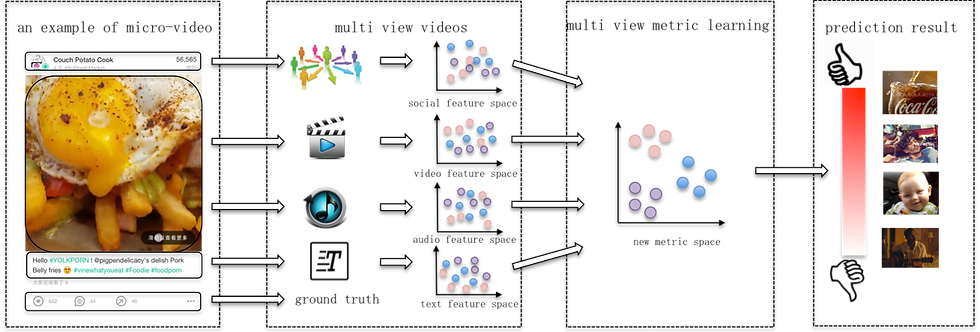
Figure 1: Micro-video popularity prediction via our proposed TMALL model.
Figure 1: Micro-video popularity prediction via our proposed TMALL model.
Considering our dataset not only contains micro-videos that have received millions of views (loops/comments/likes/reposts) but also plenty of micro-videos that receive small amount of views. To deal with the large variation in the number of views of different micro-videos, we apply the log function on the number of views. Furthermore, since micro-videos tend to receive views over some period of time, to normalize for this effect, we divide the number of views by the duration since the upload date of the given micro-video. The results are shown in Figure 2. We find that this resembles a Gaussian distribution of the view counts as one would expect.
Data Pre-processing
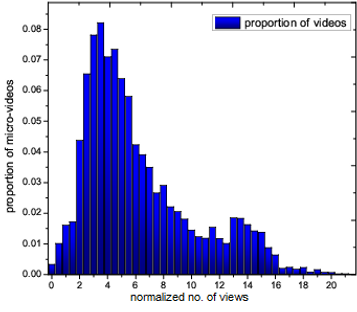